Data Observability for Financial Services
Table of Contents
FinServ's Data Challenges
Exabytes of data flow through financial services systems every day.
The rise in mobile banking, open financial data, and artificial intelligence has created increasingly complex webs of pipelines and other tools. And as the volume of data in the industry grows, companies are working to strengthen their observability capabilities.
The term ‘data observability’ refers to the ability to track, perform root cause analysis, and fix data issues in real time. Whether a cell gets deleted in transfer or a data field doesn’t match across documents, data corruption can compound as files and tables move through a company’s myriad databases and systems.
Data corruption can compound as files and tables move through a company’s myriad databases and systems.
Robust data observability prevents these errors, corrects them before they make an impact, and alerts quality assurance teams to additional issues that need resolving.
What is Data Observability?
Data observability is the collection of actionable information about data quality combined with the ability to track down and remediate issues. Whereas data monitoring can tell an organization about their data health, observability typically implies maximum coverage as well as remediation tools.
For financial services organizations—like banks, lenders, Regulators and Government Sponsored Enterprises (GSEs)—advanced tools like data monitoring, data quality, and data observability have become critical to delivering exceptional and secure digital experiences. The practice becomes even more essential against a backdrop of increased cybersecurity threats and regulatory oversight.
Read: Key Terms in Data Observability
In this blog, we’ll reveal the critical need for advanced data technology in financial services, the competitive advantages that financial institutions can create, and the ideal solution stack to wrangle, organize, clean, and extract value from data.
4 Reasons to Adopt Data Observability
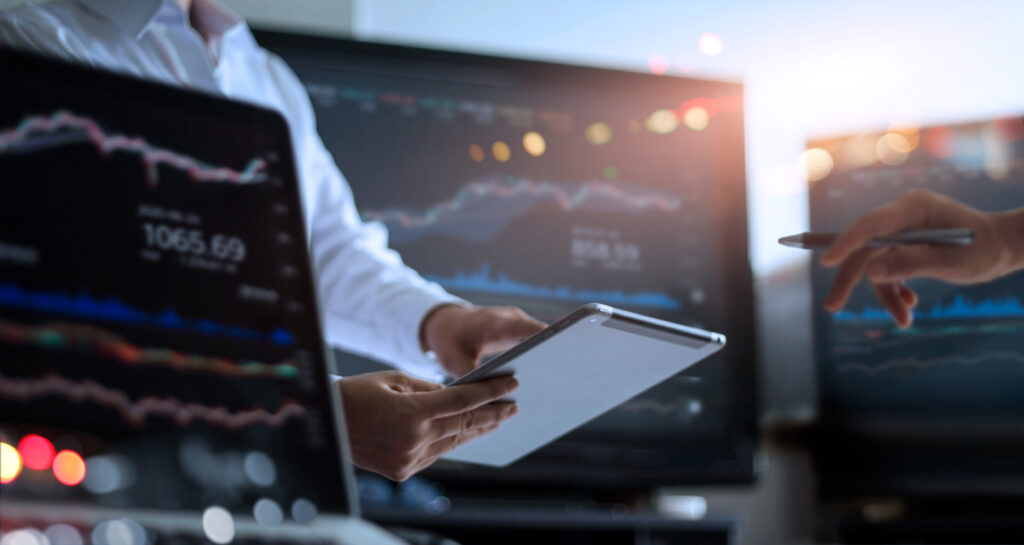
The financial services industry is increasingly reliant on data-driven decision-making. But managing all the information that fuels those decisions becomes untenable with yesterday’s technology.
Consider this: global digital banking users are expected to reach 3.6 billion by 2024—up from 2.4 billion users in 2020—according to a report by Juniper Research.
Trends like open banking and mobile-first financial services are driving this growth, while increasingly tangible advances in artificial intelligence and machine learning are turning the c-suite’s eyes toward data to sharpen and create new competitive advantages.
Here are 4 key areas where the financial services executives should investigate a future-forward approach to data observability.
Automated Quality Assurance
Preventative Data Health
Volume Management
Insight Discovery
1) Automated Quality Assurance
With every customer comes a tranche of documents, forms, records, identification numbers, personally identifiable information, ad infinitum. These files traverse a complex network of partners and applications, creating confusion around a record’s veracity or lineage.
Files traverse a complex network of partners and applications, creating confusion around a record’s veracity or lineage.
Typically, a bank or lender may hire a team of analysts to manually review data, find inconsistencies, and correct inaccuracies. This approach not only introduces human error, but also comes with deadlines that only adds unnecessary stress to the workday.
Additionally, manual reviews can’t scale with high volume, so you end up evaluating only a sample of the files in your portfolio. Peaks and valleys in volume leave financial services companies in a constant state of hiring and training, then reducing staff, which harms morale and bears risk and expense.
Data observability solutions help to create reliable financial and operational results to the costs of scaling.
2) Preventative Data Health
Healthy data engenders consumer trust and efficient compliance operations. And like nine out of ten dentists, data technologists recommend a preventative approach to maintain data health.
Data engineers use a host of tools like data monitoring, data validation, data quality management, and data observability to understand their data quality at every lifecycle stage and take action to prevent corruption.
Still, these tools are designed for engineers, and not all key executives are granted control over the data observability process. By integrating automated data validation processes with intuitive, no-code user interfaces, advanced data health trackers give total visibility to leaders across an organization.
By integrating automated data validation processes with intuitive, no-code user interfaces, advanced data health trackers give total visibility to leaders across an organization.
With preventative data health strategy, financial services organizations can safeguard their business against regulatory penalties, foster greater customer confidence, and enable better decision-making.
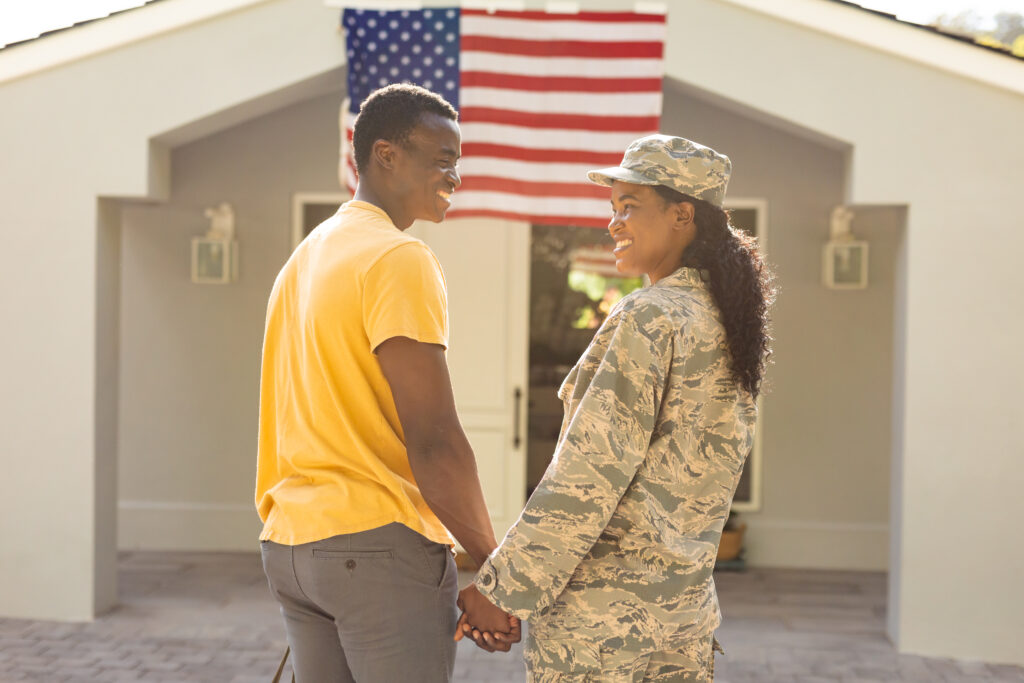
Mortgage servicers use data observability tools like BaseCap to keep track of active duty servicemembers in their portfolio, in order to meet SCRA compliance.
3) Volume Management
Managing the sheer volume of data generated by financial services is a significant challenge facing Chief Data Officers (CDO).
Tasked to optimize storage resources, enhance processing speeds, and streamline data accessibility, CDOs often turn to the next-gen solutions to accomplish their goals.
For example, Goldman Sachs, a global investment banking firm, has invested in cutting-edge data compression technologies to efficiently handle the massive influx of trading data. By compressing data without compromising its integrity, Goldman Sachs minimizes storage costs and accelerates data retrieval times, empowering traders to make timely investment decisions.
Making data available is important, but maintaining the integrity of that data is even more crucial.
The traditional data management approach does not scale easily due to heavy manual processes and financial limitations. Through automation software, organizations can break through these scalability barriers and maintain 100% visibility into their information.
The traditional data management approach does not scale easily due to heavy manual processes and financial limitations.
4) Insight Discovery
The path from data to insight can be long and winding. Companies must move information from their data sources, which often originate in an array of formats and/or must be drawn from documents, into data warehouses, through data pipelines into various visualization software, and finally to data consumers/analysts, who interpret the information into insight.
That’s a long sentence and an even longer process!
Read: Key Terms for the C-Suite
Banks and other institutions need a better way to be alerted to and act on data quality issues throughout the entire journey.
Banks and other institutions need a better way to be alerted to and act on data quality issues throughout the entire journey.
Validation, automation, and transformation tools help direct operational users to discover trends and risk priorities in their data sources. This real-time observability improves the analysis, visualization, and decision-making that organizations need to remain competitive and sound.
Thanks for reading!
Sign up for new content about data observability, automation, and more.
About BaseCap
BaseCap is the data health platform that helps operations teams prevent and correct bad data. Top US banks use BaseCap for quality control, process automation, and compliance management.
"I think the tool is great because it's an out of the box solution where you can give a business admin, or someone that's knowledgeable enough from a tech perspective and a business perspective, to really drive and make the changes and really own the administration of the tool."
Jeff Dodson, Lument