Why Data Quality Can Make or Break Mergers & Acquisitions
Table of Contents
Current State of M&A in Financial Services
Mergers and Acquisitions in the financial services sector have been particularly active in recent years. 2023 saw a notable uptick in M&A activity, with a 15% increase in deal volume compared to the previous year, according to PwC’s M&A Insights 2023.
Key drivers of this increased activity include the need for digital transformation, competitive pressures, and the desire to acquire new capabilities. For example, many traditional banks are acquiring fintech firms to enhance their digital offerings and stay competitive in an increasingly tech-driven market.
Many traditional banks are acquiring fintech firms to enhance their digital offerings and stay competitive in an increasingly tech-driven market.
The most common struggle with mergers and acquisitions in the financial sector involves data. Disparate systems, poor initial data quality, and manual data validation processes create complexity that can ultimately devalue the initiative.
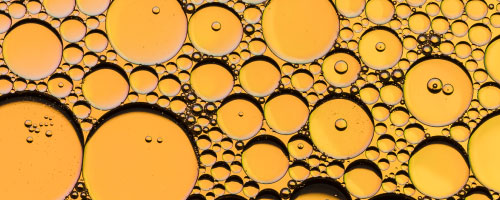
Combining loan data from the seller’s servicing system to the buyers’ platform, for instance, can be like mixing oil and water. System reconciliation software can help.
Even the same two systems can have integration challenges, as factors like naming conventions, table formats, and even decimal point placement can derail what at first seems like an easy data transfer.
Read: Simplifying Data Transfers
To prepare for your next merger, whether you’re selling your business, buying another firm, or combining strengths into a new company, data quality must take first priority. Here are some ways we’ve seen banks and lenders use data validation before, during, and after major M&A activity to extract the most value possible from the transaction.
Pre-Merge: Preparing Data Pipelines
Before embarking on an M&A journey, it is essential to ensure that the data involved is accurate and reliable. Pre-M&A data validation is critical in identifying potential data issues that could derail the process, or certifying data accuracy to support valuation decisions.
Data quality is important both for the buying organization and the business being sold. For instance, reliable reporting of high data quality can make a mortgage servicer more attractive to a larger company looking to acquire their line of business. The buyer doesn’t want to inherit someone else’s data integrity issues; often they will walk away, negotiate the value downward, or insert contractual terms that are onerous or punitive to the seller.
The buyer doesn’t want to inherit someone else’s data integrity issues; often they will walk away, negotiate the value downward, or insert contractual terms that are onerous or punitive to the seller.
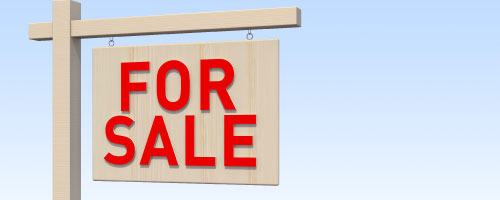
On the other hand, enterprises that are buying smaller businesses need to ensure that poor data integrity does not diminish the value of the sale. They’ll want assurances to defend their valuation stance.
For example, one BaseCap customer was able to reduce the number of full-time employees required to run quality assurance on their loan portfolio from 18 to two. This kind of process efficiency shows potential buyers that the business is running at peak margins.
Steps to validate data before M&A:
- Conduct Data Audits: Perform comprehensive audits of data and QA processes to identify inaccuracies, inconsistencies, and missing information.
- Establish Data Validation Protocols: Define clear protocols for data validation, including the criteria for data quality and the tools to be used.
- Create Detailed Reporting: Be able to demonstrate high data quality and effective quality assurance protocols in rigorous detail.
Mid-Merge: Connecting Disparate Systems
The period after a merger can easily devolve into frozen processes and severed silos. We’ve seen a seller effectively locked out of all data systems once acquired by a larger company. Factors like strict security protocols and divergent data pipelines slow down productivity to a halt.
Factors like strict security protocols and divergent data pipelines slow down productivity to a halt.
Continuous data validation during this phase is crucial to maintaining data integrity and ensuring a seamless transition. Moreover, key technologies like document recognition and data transformation must be deployed to turn unstructured data into tables that can be compared to the buyer’s system of record.
Read: Document Validation for Finserv Risk Management
For example, loan documents often include PDFs and handwritten forms. If names are written wrong on one of these documents, it can impact downstream processes and even affect the borrower. Tools like OCR allowed one BaseCap customer to convert their documents to spreadsheets, simplifying the comparison of the data. They could also use the BaseCap platform for version control to track down which files were responsible for data issues and quickly act on fixing those errors.
Steps to validate data during M&A:
- Real-Time Data Validation: Implement real-time data validation techniques to monitor data quality continuously throughout the process.
- Data Integration: Use data integration platforms to ensure that data from different sources is accurately combined.
- Regulatory Compliance: Ensure that data validation processes comply with relevant regulatory requirements to avoid legal complications.
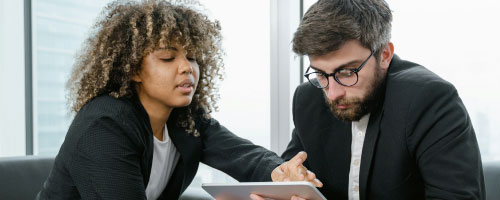
Post-Merge: Long-term Data Quality
Enterprises can accelerate the decisions they make with new assets only when core data is clean, stable, and well-supervised. In an ideal world, where the proper steps are taken before and during a merger, implementing effective ongoing data observability is quick and easy. In the worst scenarios, businesses are left with lengthy data evaluation projects that sap much of the gains promised by the deal.
Often, the greatest risk involves regulatory reporting. When the buyer obtains invalidated data, they expose themselves to external auditing or customer dissatisfaction.
When the buyer obtains invalidated data, they expose themselves to external auditing or customer dissatisfaction.
Implementing Ongoing Data Validation Processes:
- Identify Critical Data Elements: Determine which data elements are critical for business operations, compliance, and reporting. Focus on the quality of these high-priority data sets.
- Establish Data Governance Policies: Develop and enforce data governance policies that define data ownership, data stewardship, data quality standards, and data management processes. This includes setting up a data governance committee or council to oversee data-related activities.
- Leverage Automated Data Quality Tools: New technologies automate data cleansing, validation, and enrichment processes. These tools can help detect and correct data errors, standardize data formats, and ensure data consistency.
Final Thoughts
Data validation is a critical component of successful mergers and acquisitions in the financial services sector. By ensuring data integrity before, during, and after M&A transactions, financial enterprises can mitigate risks, enhance operational efficiency, and achieve their strategic objectives. As the M&A landscape continues to evolve, robust data validation practices will remain essential for realizing the full potential of these endeavors.
Thanks for reading!
Sign up for new content about data observability, automation, and more.
About BaseCap
BaseCap is the intuitive data validation platform that operations teams use to accelerate compliance and risk management processes.
"I think the tool is great because it's an out of the box solution where you can give a business admin, or someone that's knowledgeable enough from a tech perspective and a business perspective, to really drive and make the changes and really own the administration of the tool."
Jeff Dodson, Lument