Half a Billion and Counting: The Real Cost of Bad Data
Citigroup was ordered to pay more than five hundred million dollars in fines for poor loan quality.
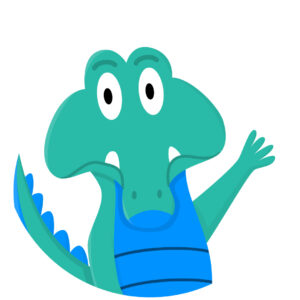
Data Gator
BaseCap's resident data expert
Citigroup was ordered to pay $135 million in fines for making insufficient progress remediating its problems with data quality management and failing to implement compensating controls to manage its ongoing risk, according to the Federal Reserve.
The action taken by the federal government conveys a clear mandate for financial institutions: improve data quality management or pay a hefty price.
The action taken by the federal government conveys a clear mandate for financial institutions: improve data quality management or pay the price.
Only four years ago, Citigroup agreed to pay $400 million to federal regulators for a similar “failure to establish effective risk management” in the business.
More than half a billion lost to regulatory fines over a four-year period. Read on to discover how companies can avoid this fate through proper data quality management.
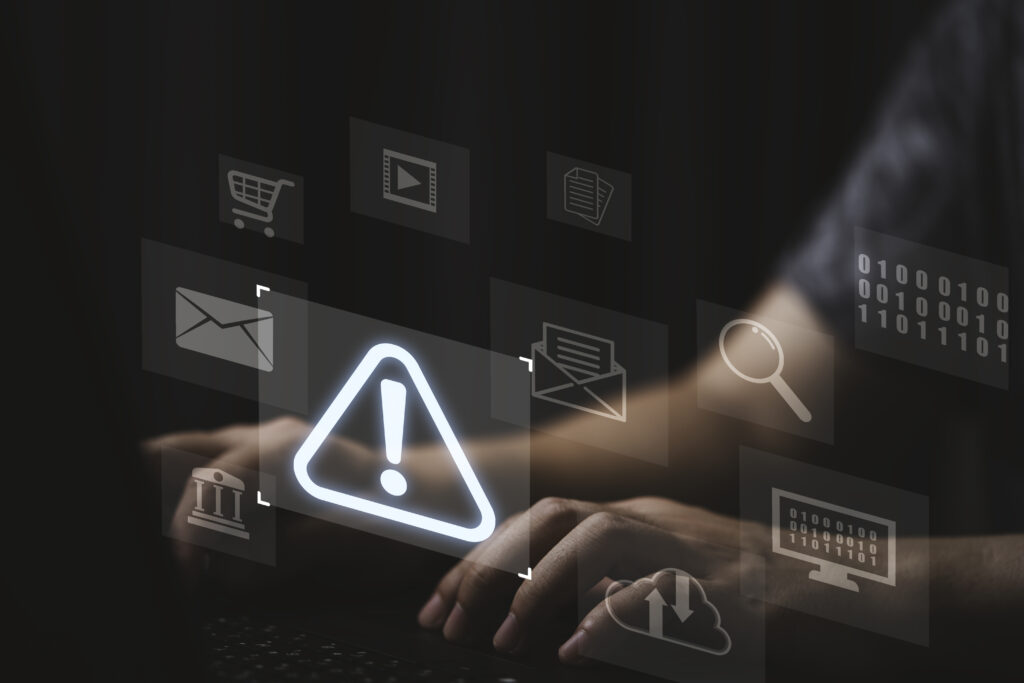
Enterprises use data validation software to improve compliance management and protect themselves from risk.
Details of the infraction
- Citigroup erroneously reported details of loans amounting to tens of billions of dollars
- The mistakes created friction between Citi and McKinsey, the firm hired to remedy issues discovered in 2020
- Citi has 30 days to create a plan to fix the loan errors and other data issues
- Commercial loan errors discovered included incorrect maturity dates, collateral, information, and even the size of loans
What went wrong?
- Chronic technology and regulatory issues have plagued Citi for years
- Employees were incentivized to address surface issues quickly, rather than find and fix root causes
- Employees were told to ignore gaps in data controls or hide them from regulators
- Regulators rejected plans created by McKinsey on behalf of Citigroup
How to avoid the same mistakes
Organizational change is difficult, in large part because it is cross-functional. Yet, businesses that embrace a collaborative approach to data quality are more likely to succeed. Just as Microsoft has emphasized ‘teamwork’ in their security strategy, organizations must rely on teamwork to improve data quality.
Better data quality collaboration requires:
Preventative data health
Most enterprises address data issues after they’re discovered. However, preventative data quality controls can reduce errors before they become issues. Consider the expense of a root canal compared to a pack of floss; data quality is no different.
Consider the expense of a root canal compared to a pack of floss; data quality is no different.
Implement solutions that help multiple teams track and prevent data issues before files enter your systems and as they move to different systems. Often, this approach is called “data observability,” and goes a long way toward eliminating missing or inaccurate data.
Automated quality assurance
Since data issues abound, and employees typically address them manually, correcting bad data can sap resources, inflame headaches…and still fail. Adding automation to QA processes helps banks and lenders speed up the discovery and remediation of data errors so they can focus on preventing them.
Manually correcting bad data can sap resources, inflame headaches…and still fail.
One way to automate data validation tasks is through an advanced rules engine. Business users can write policies with which to check their data for certain parameters, and then automatically assign data issues to the correct personnel for remediation.
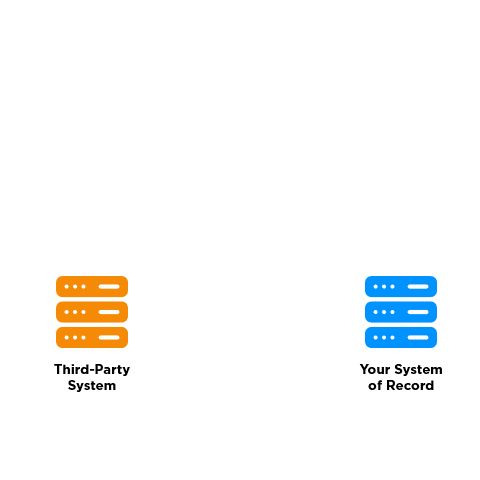
Combining document recognition, an advanced rules engine, and automation features into one platform, BaseCap is the preferred choice for mortgage lenders looking to improve their data quality.
Intelligent document processing
A large portion of data issues related to loans involves document data. PDFs, handwritten notes, tax forms, and other documents do not conform nicely with origination and servicing systems. Optical Character Recognition (OCR), the technology that extracts data from these docs, has at best a 3% error rate. Compounded across millions of data fields, 3% becomes an untenable margin.
Optical Character Recognition (OCR), the technology that extracts data from documents, has at best a 3% error rate.
Enterprises that add data validation to OCR processes, however, can eliminate errors from document recognition before the data ever enters their system.
Thanks for reading!
Sign up for new content about data observability, automation, and more.
About BaseCap
BaseCap is the intuitive data validation platform that operations teams use to feed quality data to AI algorithms.
"I think the tool is great because it's an out of the box solution where you can give a business admin, or someone that's knowledgeable enough from a tech perspective and a business perspective, to really drive and make the changes and really own the administration of the tool."
Jeff Dodson, Lument