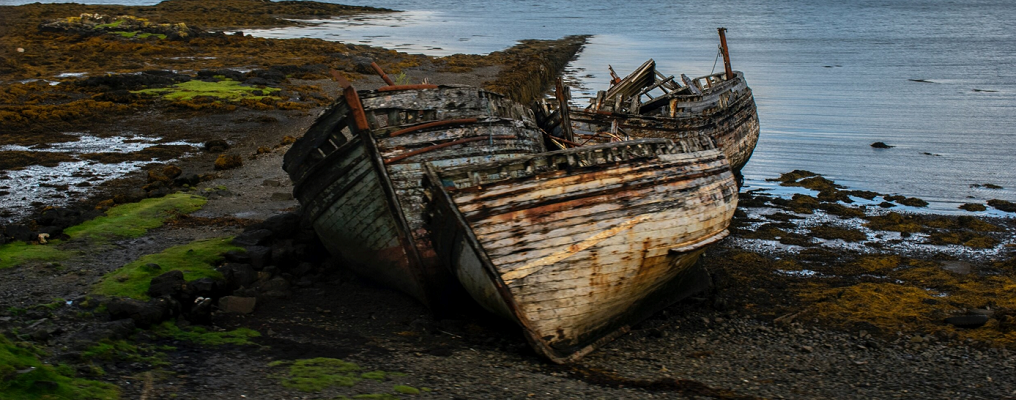
The Increasing Dependence on Data
Organizations across industries are becoming more “data-driven.” The mortgage industry for example, faces downward margin pressures and immense competition from a surge of fintech upstarts. Some are turning to Artificial Intelligence/Machine Learning (AI/ML) models to identify new opportunities, such as launching campaigns for clients who may want to refinance their mortgages. However, for these initiatives to be effective, data quality is paramount. Poor data quality may lead to ineffective campaigns and other detrimental consequences.
Consequences of Poor Data Quality
Most data governance and data quality programs only cover the “tip of the iceberg.”
Harvard Business Review (HBR) estimated that companies in the U.S. experience a $3.1 trillion annual cost related to bad data, and that knowledge workers spend about 50% of their time addressing data issues. Also, at an aggregate level, 30% of annual revenue is lost to bad data. (Entrepreneur.com)
There are four big reasons why poor data quality is such a significant revenue and resource sink.
1. Unscalable Growth – Leading to a Loss of New Business Opportunities
An investment firm significantly expanded its mortgage portfolio resulting in a reliance to source data from a growing list of loan servicers.
-
The growing amount of data meant more business opportunities, but it also meant the firm had more data quality issues to address. Although refinance volume hit a record high of $867 billion for Q3 2020 (Black Knight), the firm’s staff was burdened by the time-consuming process of triaging the data, conducting root cause analysis, remediating, fixing and validating the fixed data manually.
-
Poor campaign results were impossible to diagnose as models were applied to a flawed underlying dataset. Are faulty results related to the logic in the model or to the underlying data?
-
The manual process to address data issues delayed liquidations and caused inaccurate risk assessments, leading to lost revenue. Even more damaging, poor data quality prevented timely insights for key decision making, dragging the firm behind its competitors in forging new opportunities.
2. Unsustainable Operations – Inefficient Operating Cost
The same firm considered several approaches to address growing data issues in order to continue expanding its business.
-
Hire data engineers and specialists with data science expertise, but lacking the domain knowledge required to address mortgage-related data issues.
-
Shift capacity from analysts who were domain experts in the mortgage field but lacked the depth in data science to effectively address data issues.
-
Hire external resources that are costly and not operationally sustainable.
The firm also considered different combinations of these approaches, but they all involved increasing costs as the amount of data grew. In other words, its roadmap for portfolio growth was going to result in a further decline in margin.
3. Deflection of Responsibilities – Corrosion of Team Cohesion
Data issues have also created friction within organizations, damaging team chemistry on top of impacting the bottom-line.
-
A loan originator started to see an uptick in loan buybacks because of increasingly poor risk assessment. Bad data was the culprit, and each department pointed their fingers upstream. With so much blame to go around, the company’s collaboration, effectiveness, and morale was in decline.
-
Subsequently, due to increasing error rates, employees started to question the decision making of managers, or the information coming from another department or source. The lack of confidence in their data led to increased interruptions. More conference calls and fire drills encroached on full schedules.
4. Diminished Perception of Company’s Branding, Customer Loyalty, and Regulatory Oversight
Data issues have also contributed to externally facing problems that may be even harder to remediate than those within an organization:
-
Bad data delayed a firm’s loan processing rate and its partners and customers had taken notice. The damage to a firm’s reputation was not easy to repair. Not only did the firm need to resolve its data quality issues, it needed to spend marketing dollars to repair its reputation and catch up to competitors who gained an edge by effectively adopting data quality controls.
-
A SIFI bank recently had to adjust to a new capital calculation methodology to remain in compliance with the latest regulations. Inaccurate source data led to erroneous calculations and reported results, causing the bank to misrepresent its capital risk. Fortunately, manual adjustments were made, and the company avoided regulatory penalty, which have typically been made public. However, this required the added expense of hiring a consulting firm to redesign the data pipelines and update the bank’s data models. It also required existing bank staff to dedicate more hours to remediation capacity, causing morale issues.
The Key is to Nip Data Quality Issues in the Bud
The impact of data issues grows exponentially as they move down the data pipeline. This concept is captured by the “1-10-100 rule” developed by Labovitz and Yu. Essentially, the cost of fixing data will balloon as bad data moves downstream to the use cases such as analytics, data modeling, and reporting. Today, this concept remains demonstrably true, so it is critical that organizations focus on establishing strong data quality management that starts early in the data pipeline to prevent more impact.
Automating Data Management
There have always been a lot of inefficiencies in the process of arriving to reliable data, especially for businesses using its own staff to address data quality issues. BaseCap’s Data Quality Management Platform offers a solution that:
-
Provides an automated data quality check throughout the data pipeline and for any scale.
-
Checks and reports on the quality of the data based on business rules specific to the organization.
-
Offers insight on remediation approaches and empowers existing, non-technical staff, to collaborate on addressing data issues.
-
Allows businesses to grow sustainably, without adding more staff to manage the growing data sets that come with an expanding business.
BaseCap’s data experts have successfully helped organizations improve data management, optimize ETLs, and architectural design, maintaining a robust data quality process. By dealing with data quality issues before they ever get into the system, BaseCap has given organizations the confidence to forge forward and support a sustainable growth model.
In 2020 for example, BaseCap helped a client save $2M per year on operational costs while increasing their mortgage portfolio without additional staff. This positively impacted not only the bottom line but also the morale of the client’s analysts who were subsequently able to focus on growing their business instead of being burdened with addressing data issues manually.
While BaseCap has an initial focus on the financial services industry, its use cases can span to any industry; including healthcare, insurance, and retail.
See the Data Quality Management Platform in Action!
Schedule a demo with BaseCap Analytics, and a data expert will help design a customized approach to turn your data into a competitive advantage for your company.