The ability to extract data from documents has forever changed how businesses ingest, track, and validate critical information.
Before Optical Character Recognition (OCR)
Before the digital age, data was entirely collected on physical formats such as an application form or an invoice. To compare this data to other sources of information, like an excel spreadsheet, analysts manually extracted and entered fields through a “stare-and-compare” process. They would also manually sort, index, and store the documents.
While digitization has transformed this process for some applications, like an online application for a mortgage, much of the data being captured is still being produced from physical formats. Many companies such as those in finance and healthcare still rely on clients submitting data via paper documents.
What is OCR technology?
Early applications of OCR technology tackled issues for the blind and visually impaired. An OCR application would capture documents and convert them to either telegraph code or an audio output. For example, the post office used OCR technology to automatically read the addresses of millions of mail parcels that travel through processing centers every day.
Functionally, Optical Character Recognition follows three key steps:
1. Pre-processing
2. Text recognition
3. Post-processing
Pre-processing involves scanning the document and using various techniques to make the image easier to read.
Text recognition is then performed. There are many software programs available that will recognize the written characters and translate them into a digital format, such as XML.
Post-processing applies linguistic logic to improve the accuracy of the digital translation, improving accuracy and filling gaps based on contextual clues.
There are a wide variety of use-case scenarios where specific optimization techniques could be employed to further enhance the quality of the data captured.
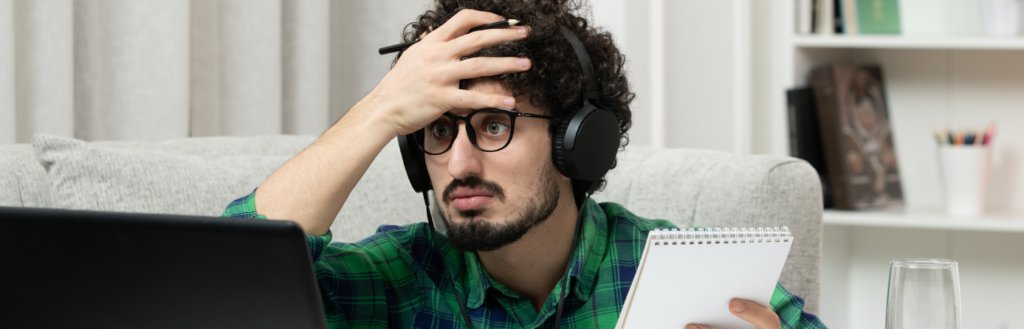
Despite great advances in OCR technology, the results often contain inaccuracies. Learn more about the 3% OCR accuracy gap.
How businesses use OCR
It’s not only the visually impaired or the post office that benefit from OCR technology. Many industries rely on data that has been captured manually from documents. OCR has the potential to improve operational efficiency by increasing the volume of data captured at any given time and eliminating human error in data extraction.
Here are three examples of how some industries benefit from utilizing optical character recognition:
Mortgage and Finance
OCR technology provides efficiency gains in loan processing, sorting, storage, and access. Loan origination expenses have been trending up – $7,452 in 3Q 2020 vs an average of $6,566 in 3Q 2008. (Mortgage Bankers Association, Dec 2020). The manual processing of these documents (loan applications and support docs such as pay stubs, bank statements etc.) is a part of this expense that can be reduced by implementing OCR to extract data. In this way, OCR technology is a weapon against the rising cost of loan origination.Healthcare and Medical – Hospitals undergoing digital transformation often leverage the nursing staff to complete data entry tasks before the end of each shift. Unsurprisingly, busy and burnt-out nurses are not the ideal candidate for flawless data input. OCR technology helps hospitals improve the accuracy of data that enteres their systems, while avoiding decaying morale for their most important staff. In fact, in one instance, “Eight people using the OCR technology dealt with an average of 10,000 PDFs per day, and the average document was 10 pages long. Each document only took seven seconds to process” (information-age.com).
Government – The United States government also leverages OCR technology to streamline data collection. State agencies, for example, use document recognition to read tax returns and dramatically reduce processing costs by as much as 70 percent and shrink the data-entry labor-force by as much as 60 percent (govtech.com). Applied across hundreds of millions of tax returns, police reports, and license applications annually, these substantial benefits multiply.
Common OCR Challenges
Despite the efficiency gains produced by optical character recognition, some organizations still do not trust the technology with their most important data. To their credit, OCR does face challenges regarding the accuracy and completeness of the output data.
OCR-captured results are usually fraught with errors due to unrecognizable handwriting and non-standard forms. Optimization techniques such as running multiple OCR engines, and enhancing pre-processing techniques can improve results, but there will always be data quality issues. Data analysts will still have to clean and remediate the output before using the captured data to generate reports, develop strategies, calculate risk etc.
However, this manual review still introduces human error.
While OCR with manual data cleanup is still an improvement on the traditional data entry approach, it can be significantly stronger if it was also paired with an automated data quality management tool.
Data Quality Manager as the Foundation
BaseCap Analytics’ Data Quality Manager is a perfect complement to Optical Character Recognition technology.
With our Doc2Data solution, a data analyst can optimize the benefits from data capture by significantly reducing the cost and effort required to clean and validate the data extracted from OCR. This can provide a boost to the near-term bottom-line and more importantly a foundation for long-term scalability of a business, which will invariably encounter more data as it grows.