Key Terms in Data Observability
Table of Contents
New to data observability?
If your job involves working with IT and operations teams to answer questions about your company’s data, then you’ve likely come across a glossary of terms like “data quality,” “data monitoring,” and “data governance.”
To the DevOps neophyte, these important data management practices may seem like one giant Venn diagram. But for operations personnel and C-suite leaders, diving into the world of data technology can often turn up more questions than answers.
Read: Data Observability for Operations
So we’ve made this helpful guide to data terminology, just for you.
Data Governance
High level management of the availability, usability, integrity, and security of the data employed in an organization.
Key roles: Chief Data Officer, Data Governance Manager, Compliance Officer, Risk Management Officer
Challenges: As the complexity of data systems used by an enterprise increases (often through natural growth, mergers, or acquisitions), balancing data security and access often becomes the first roadblock to discovering insights.
Data Lineage
Tracking the origin and movement of data throughout its lifecycle. Data lineage helps in understanding where data comes from, how it’s transformed, and where it’s used.
Key roles: Data Governance Team, IT/Engineering Team, Business Analysts
Challenges: Tracking down a data issue, like a corrupted data field or missing information, requires a high degree of coordination and collaboration between different teams and technologies.
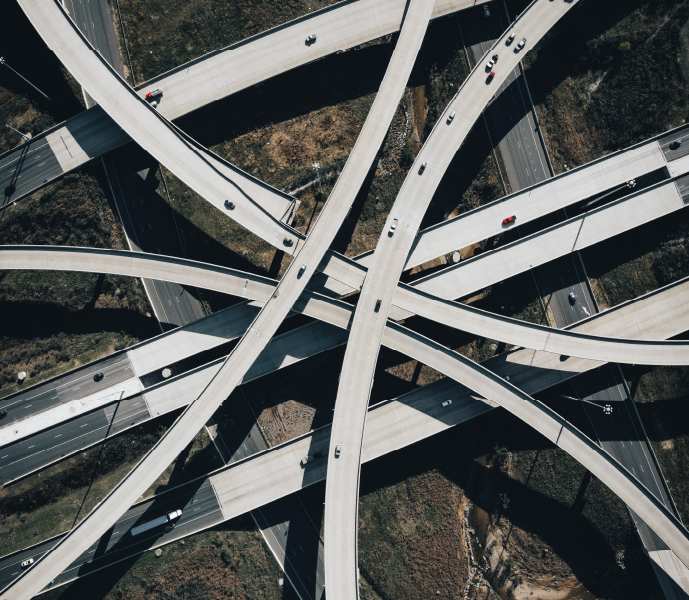
Data Profiling
The process of examining and analyzing data to gather information about its quality and structure. Structure discovery performs mathematical checks on the data to ensure phone numbers have the correct number of digits, content discovery looks into specific data fields in a spreadsheet to discover if a phone number has an area code or not, and relationship discovery identifies references between cells or tables in a spreadsheet, like connecting a cell and home phone number to a specific customer.
Key Roles: Data Governance Team, IT/Engineering Team, Business Analysts, Compliance Managers
Challenges: Proper data profiling makes an immense impact on downstream processes; however, operations teams are often too removed from the process to provide critical input into how data should be formatted, structured, and interrelated.
Data Cataloging
Creating a comprehensive inventory of all available data assets within an organization, including meta data like data types, sources, and relationships. Proficient data cataloging supports data-driven decision making by enriching information with important technical and business data, while providing a central repository for all data information.
Key Roles: Data Management Team, Chief Data Officer, Business Analysts
Challenges: Organizations typically face hurdles in finding all of their data and driving adoption of the catalog, which can slow down analytics and insight discovery.
Data Remediation
This refers to the process of identifying and resolving issues related to data quality, consistency, or integrity.
Key Roles: Chief Data Officer, Internal Auditor, Marketing Analyst, Quality Assurance Team, Compliance Managers
Challenges: Weak data governance, limited resources, and unclear responsibilities often lead to the inability to quickly and correctly remediate data issues when they’re found.
Data Security
The practice of implementing processes and technologies to protect data from unauthorized access, use, disclosure, disruption, modification, or destruction.
Key Roles: Chief Security Officer, Compliance Managers, Risk Management Teams
Challenges: Enterprises must protect their data from myriad attack vectors, from physical breaches to phishing and ransomware attacks, while still providing business teams with access to that information. Often, one goal supersedes the other, either creating vulnerabilities or preventing insight discovery.
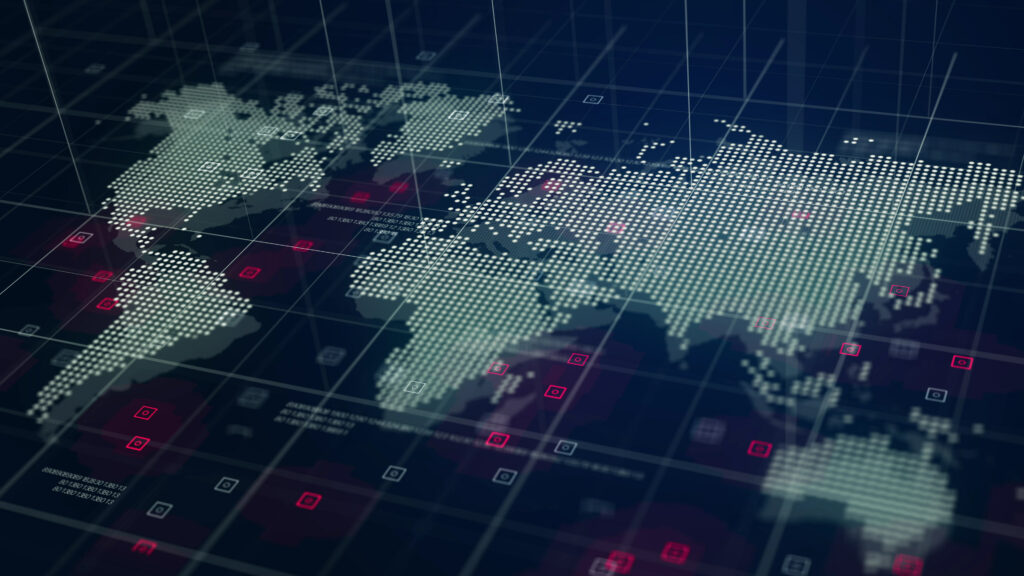
Data Monitoring
The real-time monitoring of data pipelines, or “data flows,” to detect and address issues such as latency, bottlenecks, or failures. Once created by a person or process, data tables travel through pipelines into warehouses, where they’re stored for access by business intelligence applications and AI tools. Only then does data become ready for consumers like analysts and auditors. Effective data monitoring tracks this entire journey and delivers data health “vitals” to quality assurance teams.
Key Roles: Chief Data Officer, Data Quality Manager, IT, Quality Assurance Teams
Challenges: This approach to data quality management requires significant investment in both technology and talent. It also merits a cultural shift at the organization, where the fidelity of the information feeding business growth takes higher priority.
Data Anonymization & Masking
The act of removing or modifying personally identifiable information from datasets to protect the privacy of individuals. To comply with regulations, user agreements, and SLAs, organizations must anonymize data being used for analytical purposes or machine learning models. Similar to data anonymization, data masking involves replacing sensitive information with fictitious but realistic data to protect confidentiality.
Key Roles: Chief Data Officer, Data Controller, Compliance Officer
Challenges: Anonymizing or masking data is intensely manual. Plus, without proper data cataloguing, Orgs typically have low confidence that they were able to capture all personally identifiable information.
Data Archiving
The process of transferring data that is no longer actively used to a separate storage location for long-term retention, often for compliance or historical purposes.
Key Roles: Chief Data Officer, Risk Manager, IT
Challenges: Data archival requires sizable storage space which can increase cost of ownership. It can also be difficult to retrieve old data with modern systems as technology evolves.
Data Stewardship
The responsibility of overseeing the use of data assets within an organization, including ensuring compliance with regulations and policies.
Key Roles: Chief Data Officer, Chief Technology Officer, Chief Information Officer, Data Consumers
Challenges: Because data touches so many different people and systems, data stewardship becomes a common effort across the entire organization.
Data Transformation
Converting data from one format or structure to another, often as part of the data integration or ETL (extract, transform, load) process. Transformation helps data in different files and systems speak to each other. Common transformations include a “split,” where an address held in a single cell gets separated into individual cells for street, state, city, zip. Or, entire columns can be mapped between spreadsheets despite different naming conventions or structures.
Key Roles: Data Analyst, Quality Assurance Teams
Challenges: Often, transformation requires deep technical knowledge or specialized software to accomplish, making it difficult to scale.
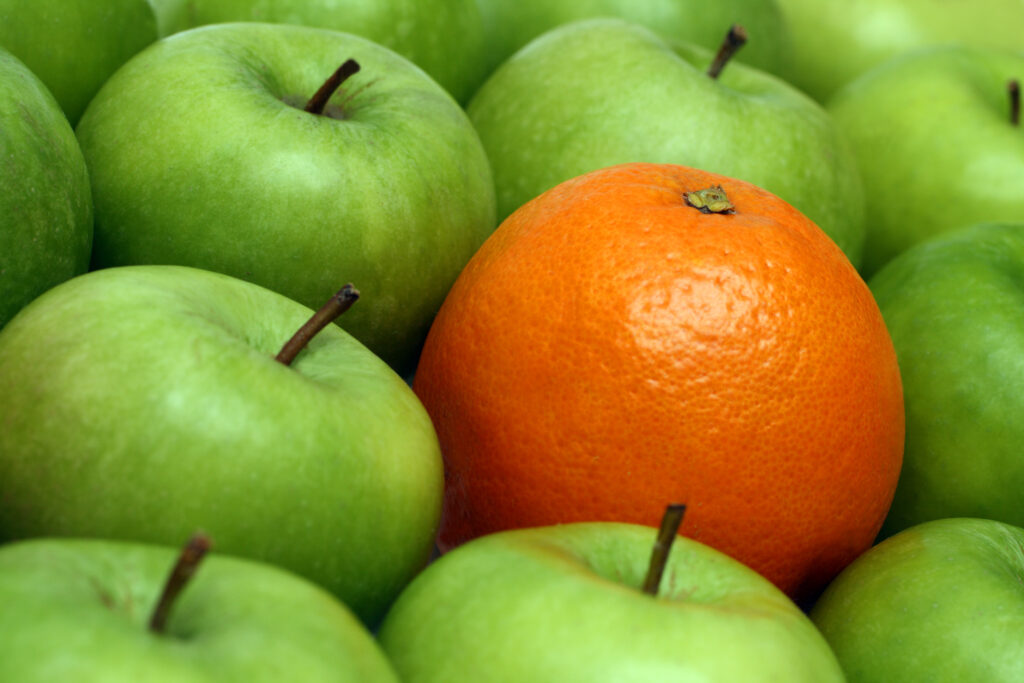
Data Mining
The process of discovering patterns, correlations, or other useful insights from large datasets using techniques from statistics, machine learning, and artificial intelligence.
Key Roles: Data Analyst, Data Mining Specialist
Challenges: Data quality and complexity can vary greatly in vast data lakes, making the insight gained from mined data dubious.
Data Retention
This involves defining policies and procedures for determining how long different types of data should be retained and when it should be deleted or archived.
Key Roles: Chief Data Officer, Risk Manager, Compliance Teams
Challenges: Typically businesses retain too much information, increasing cost of ownership as well as exposure to audits and litigation. Thoughtful data retention strategies coupled with strong archiving capabilities can help reduce these risks.
Data Privacy
Practices and regulations governing the collection, use, and disclosure of personal information, aimed at protecting individuals’ privacy rights.
Key Roles: Chief Executive Officer, Chief Data Officer
Challenges: Like data security, data privacy requires robust cataloguing, monitoring, and anonymization capabilities.
Data Telemetry
Remotely measuring and transmitting data from one location to another, often over a network. Telemetry has many applications, from the internet of things, where smarthouses transmit temperature readings to the cloud, or in data monitoring tools, where information about data quality is sent to and visualized on a dashboard.
Key Roles: IT, Quality Assurance Teams
Challenges: A standard way to remit information, telemetry does present security flaws if unencrypted and can be difficult to scale as data volume and complexity grow.
Data Observability
The collection of actionable information about data quality combined with the ability to track down and remediate issues. Whereas data monitoring can tell an organization about their data health, observability typically implies maximum coverage as well as remediation tools.
Key Roles: Chief Data Officer, Chief Technology Officer, Chief Information Officer, IT, Quality Assurance Teams
Challenges: A standard way to remit information, telemetry does present security flaws if unencrypted and can be difficult to scale as data volume and complexity grow.
Data Health
A fun marketing term used to describe the combined quality, accessibility, security, and observability of a company’s data.
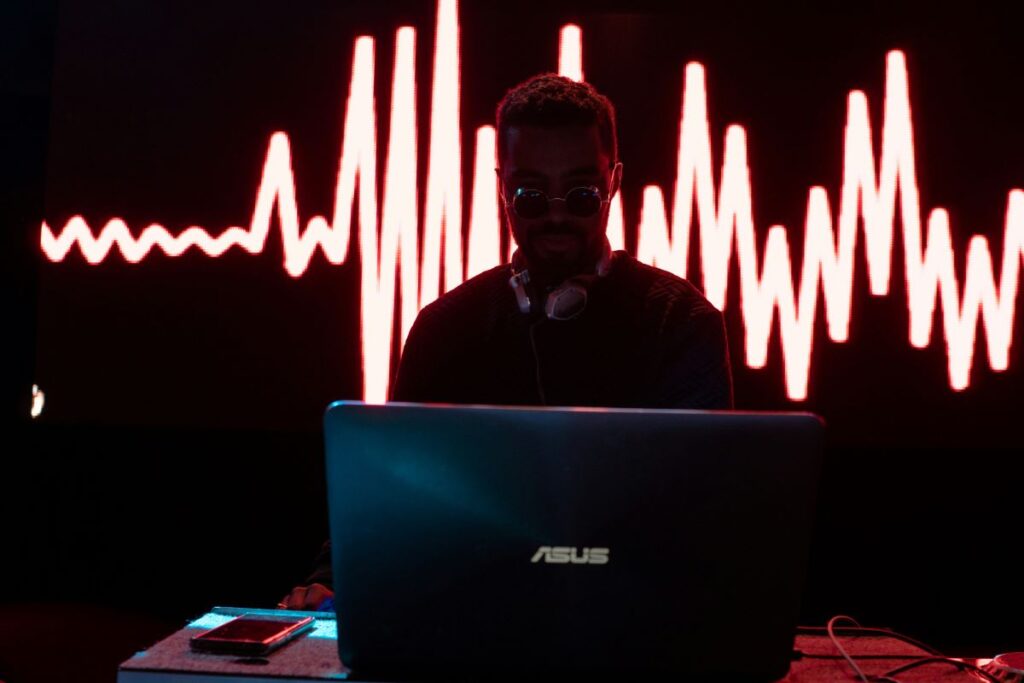
Thanks for reading!
Sign up for new content about data observability, automation, and more.
About BaseCap
BaseCap is the intelligent data health platform that lets business users prevent and correct bad data. Top US banks use BaseCap for quality control, process automation, and compliance management.
"I think the tool is great because it's an out of the box solution where you can give a business admin, or someone that's knowledgeable enough from a tech perspective and a business perspective, to really drive and make the changes and really own the administration of the tool."
Jeff Dodson, Lument